How accurate are drone mapping surveys?
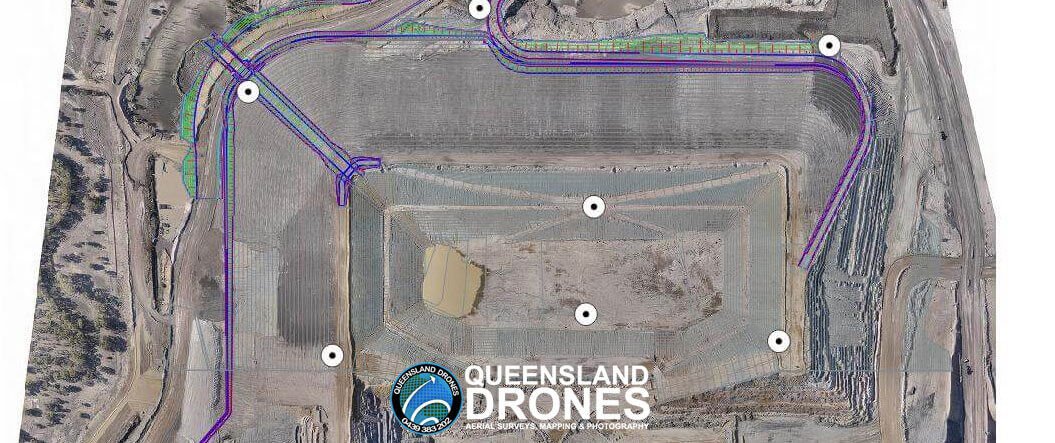
Can Drone Aerial Surveys Meet Engineering Standards?
Drone mapping surveys are being used more and more to support construction, development, mining, agricultural and other commercial activities. Queensland Drones is a trusted provider of precision PPK Precision Aerial Mapping using purpose-built mapping UAVs. We regularly partner with engineers, surveyors and other industry professionals to rapidly deliver survey-grade aerial mapping (especially over larger areas).
So how accurate are aerial mapping surveys using drones? And why does experience matter so much?
It’s pretty easy to buy a drone and fly a site for mapping data. You can process it on an online platform like Pix4D or DroneDeploy and get what looks like fairly good outputs. You can even buy something called Aeropoints that will provide a level of ground control for additional accuracy.
In fact, lots of drone operators think that’s all you need. And some construction companies think the same. But is that enough? And if so, why do US survey authorities insist on aerial mapping being delivered only by licensed surveyors (even if Australian authorities don’t)?
Survey accuracy and reliability are critical if you intend to use the data for engineering or construction purposes. Basing your project on flawed data can lead to major problems and legal liability issues – something most drone operators know nothing about.
There are situations where drone imagery is all you need to inform your project planning or pre-design work, but before you rely on drone-based map to plan earthworks, building foundations or other structural and engineering uses where accuracy is critical, you need to know they can be certified by a registered surveyor.
When we create aerial survey maps, we use industry-standard GIS-grade PPK and RTK survey equipment to level and align the photogrammetric data for maximum accuracy. Where ultimate precision is required, we use ground control points and checkpoints measured by a qualified surveyor.
Some drone operators don’t use PPK/RTK or GCPs at all, but rely entirely on a 3rd party processing platform like DroneDeploy or Pix4D to provide the accuracy. These platforms provide pretty outputs, but not accuracy, and there is literally no way to measure how accurate or inaccurate the results may be. It’s not worth the risk.
But to understand that fully, we need to talk about what accuracy really means.
What does “accuracy” mean for drone survey mapping?
There are two types of accuracy when it comes to drone mapping surveys (or any other kind of survey) – Relative Accuracy (also known as local accuracy) and Absolute Accuracy (also known as global accuracy).
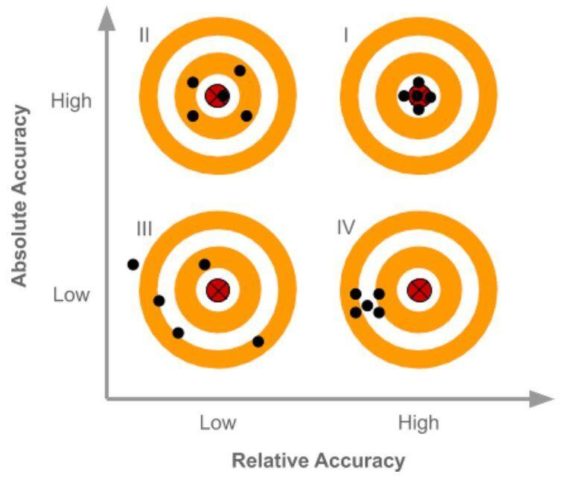
What is relative accuracy?
Relative Accuracy (also called local accuracy) reflects only how accurate the position of one pixel, position or object in the model is to another pixel, position or object in the model. You could measure absolute accuracy with a tape measure or ruler.
High relative accuracy can be useful on it’s own, if the model does not need to be fitted into a geospatial framework. But maps with low relative accuracy and low or no absolute accuracy are just pretty pictures, and should be treated as such.
It’s useful to understand that an “off the shelf” consumer drone like the Phantom 4 Pro or the Mavic Pro relies on public satellite data for its precision, and that data is often only accurate to 3-5m (yes metres) horizontal, while vertical accuracy is almost meaningless. So if your mapping operator is using a drone like this, they are not doing you any favours.
Find out more about relative survey accuracy
What is absolute accuracy?
Absolute Accuracy is a measure of how accurate the latitude, longitude and elevation (or northing, easting and height) of a pixel, position or object in the model are in comparison to their real world position if measured by a survey instrument.
The only accurate way to measure the absolute accuracy of aerial mapping data is with the use of checkpoints (ground control points measured using survey instruments, but not included in the processing of the model). Checkpoints need to be independently measured by an authoritative source.
We have had the absolute accuracy of our final mapping products measured by registered surveyors at 1.5-2cm horizontal, 2.5-4cm vertical, when based on PPK data collection accuracy combined with surveyed ground control points and checkpoints.
Find out more about absolute survey accuracy
How is accuracy measured in drone mapping surveys?
The only established method of checking and proving the accuracy of drone mapping surveys is using independently measured checkpoints.
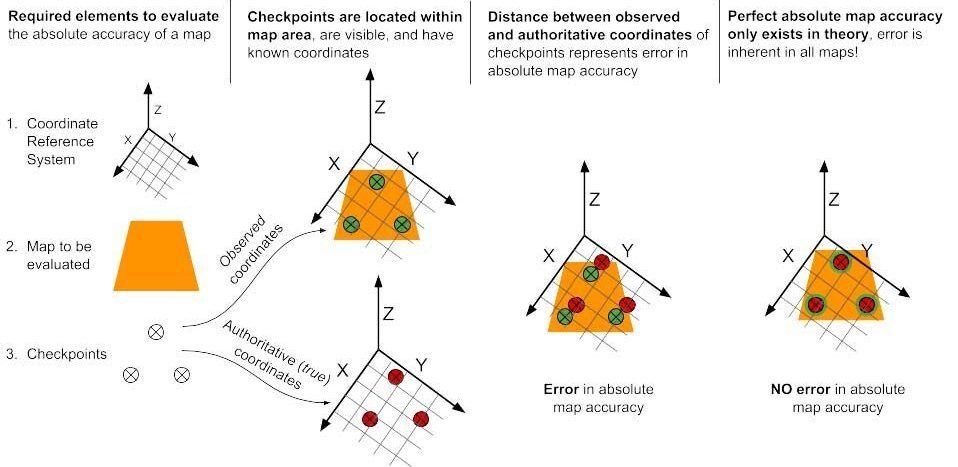
Absolute accuracy in drone survey data is measured by comparing the observed coordinates (the positions of checkpoints in the model) against authoritative positions (the independently measured coordinates of the same points on the ground).
How accurate should drone mapping surveys be?
There is no such thing as perfect accuracy in drone mapping surveys (and there is contention regarding the extent to which perfect accuracy may exist in traditional survey models). Every step in the process of collecting the data and creating the outputs potentially adds a small error factor to the end result.
The first element that needs to be considered in estimating the precision of drone survey data is the Ground Sampling Distance (GSD) – a measure of the smallest object which would be visible in the original photos, before they are processed into mapping outputs.
For example, we often fly the Swiss-made Wingtra One PPK UAV for highest survey data accuracy, as it’s 42MP mapping camera is capable of achieving a Ground Sampling Distance of 5-8mm per pixel, depending on terrain and flight altitude. This means an object the size of a 5c piece could be detected in the photos.
GSD is important because it affects the minimum relative accuracy possible within the model, which itself affects the minimum absolute accuracy. If the data is collected, for example, with a Phantom 4 Pro UAV at 120m altitude, the GSD will be about 2.6cm/pixel at best. In practice, this means the best possible relative accuracy in the model would be 5.2cm horizontal and 7.8cm vertical – simply because that’s the closest a person or system could get to identifying the centrepoint of a GCP or checkpoint in the images.
But GSD is only ever relative to the precision of the mapping camera and the height of the UAV above the terrain. The extent to which the UAV is able to follow or reflect changes in the terrain will determine the overall GSD (and therefore the overall relative accuracy) of the model.
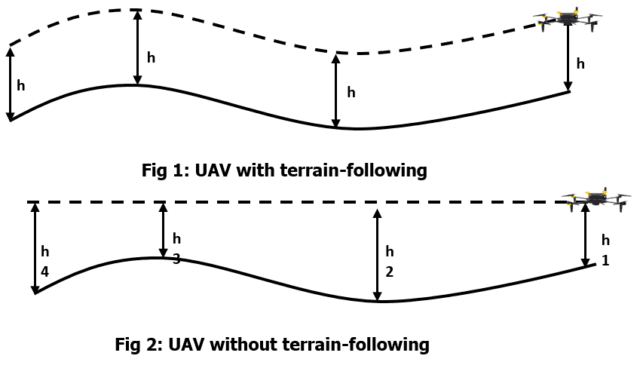
Most UAVs these days feature some kind of terrain following capability. But sadly, few do it well. Most will establish a waypoint at each end of a grid and will adjust the altitude for those two points. If one point is low (say 100m above sea level) and one point is high (say 200m above sea level), the drone will gradually climb or descend between those two levels – ie, if set at 120m flight altitude, the drone may start a grid line at 220m high and end at 320m high, but will not reflect any variance in the terrain between those two points (e.g. there might be a gully 50m deep in the middle). While the GSD at the start and end of the grid line might be 2.6cm/px, over the centre of the gully it might be 4cm/pixel or more.
It is not uncommon in rugged terrain for a survey model configured to be executed at 1.5cm or 2cm per pixel GSD to end up with 15-20cm/pixel final GSD simply due to elevation changes during flight. The only way this can be effectively corrected is using ground control points so the processing system understands the changes in the terrain during flight.
What is survey-grade accuracy?
The term “survey grade accuracy” (sometimes called GIS-grade accuracy”) has no absolute definition, so you have to be careful about accepting this as a quality statement. In general, it implies an absolute accuracy of 10cm or less. But surveyors would argue that engineering-grade surveys should be accurate to within 3cm or less.
In reality, “survey grade accuracy” is what ever the client believes is the minimum error which can be managed in the data. There are really very few cases where 3cm accuracy is an absolute requirement (as distinct from a “nice to have”). It is achievable, but everything comes at a cost.
The larger the mapped area, the less the error in relative accuracy is critical to the outcome. For example, if we assume that relative accuracy is one metre for a given aerial mapping technology and we’re measuring 25km of fence line, an error of 1m or so is not terribly important. But if we’re measuring a retaining wall 10m long, an error of 1m is pretty significant.
What is RMS error in drone mapping surveys?
RMS error is the final calculation of the accuracy of the outputs from drone mapping surveys when all possible sources of error have been factored into the model (not just GSD or GPS error). There are many ways that small accuracy errors can be introduced into a drone mapping survey. For example, if the centre of a GCP is a line 2cm wide (so it can be easily identified in the photos), then positioning that point in the imagery will have a potential error of 1-2 cm just because of the thickness of the line. Add this to the positional accuracy of PPK or RTK (typically 1-2cm at best) and you have a total potential RMS error of 4-6 cm already.
Drone maps created using good quality drone equipment, careful flight planning, commercial grade GPS ground control points and commercial grade processing software can potentially be accurate to around 2-3cm horizontally and around 5-6cm vertically. Because we prefer to err on the side of caution, we claim our aerial mapping accuracy as <10cm horizontal and <15 cm vertical, which is generally sufficient for most purposes.
Final RMS error is calculated by establishing the X, Y and Z variance between points in the model and points on the ground.
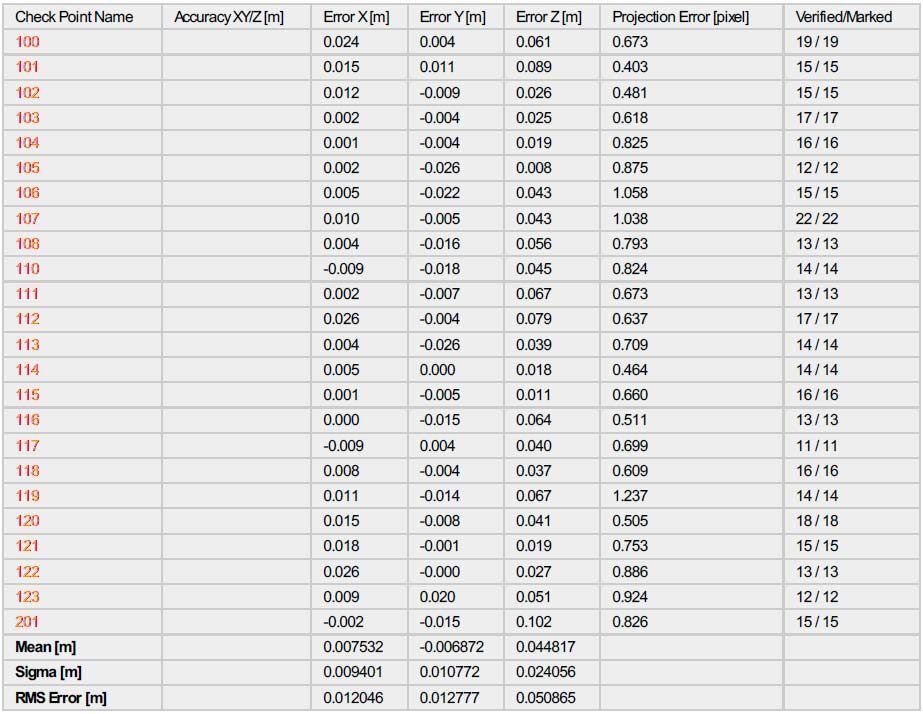
The table above shows data from drone mapping surveys carried out using the French-made Delair UX-11 mapping UAV with 24 independently surveyed checkpoints. It illustrates an overall accuracy of just 12mm horizontal and 51mm vertical. When you think that the surveyor would have struggled to achieve 6mm horizontal precision in the surveying of the checkpoints, this level of final precision is very impressive.
Note always that drone operators are not surveyors. While our mapping can be created at very high levels of accuracy, it should be certified by a surveyor (yours or ours) before being used for engineering purposes.
Contact us for an obligation-free discussion and quote for precision aerial mapping.
Comments are closed.
9 Comments
Drone mapping is a fantastic technology that has come a long way in recent years. It’s great to see useful information freely available and it will become more important as more industries adopt drone surveying and mapping
very nice article.. i learn a lot information.. thanks
Thank you for sharing your info. I truly appreciate your efforts and I will be waiting for your further post thank you once again. Henry Mickolick
Finally some truths about the ‘claimed accuracy’ of drone mapping from drone app companies with vested interests in promoting drone mapping to the masses. The truth is if you need to rely on quality data, then engage a licensed and qualified surveying or geospatial professional with experience to advise you on what you actually need to meet your unique project requirements.
This is cool, thanks for sharing. 🙂